More is different.
God is in the details.
NEWS
テンソルネットワークをベースとした生成モデルの新しい構築法を提案しその有効性を示した研究に関するプレスリリースを行いました。
- Title
- Tensor tree learns hidden relational structures in data to construct generative models
- Author
- Kenji Harada, Tsuyoshi Okubo, Naoki Kawashima
- Abstract
- Based on the tensor tree network with the Born machine framework, we propose a general method for constructing a generative model by expressing the target distribution function as the amplitude of the quantum wave function represented by a tensor tree. The key idea is dynamically optimizing the tree structure that minimizes the bond mutual information. The proposed method offers enhanced performance and uncovers hidden relational structures in the target data. We illustrate potential practical applications with four examples: (i) random patterns, (ii) QMNIST handwritten digits, (iii) Bayesian networks, and (iv) the pattern of stock price fluctuation pattern in S\&P500. In (i) and (ii), the strongly correlated variables were concentrated near the center of the network; in (iii), the causality pattern was identified; and in (iv), a structure corresponding to the eleven sectors emerged.
- Comments
- 10 pages, 3 figures
- Citation
- Kenji Harada, Tsuyoshi Okubo, and Naoki Kawashima, Machine Learning: Science and Technology 6 025002(2025)
- DOI
- 10.1088/2632-2153/adc2c7
- Code
- Adaptive Tensor Tree Generative Modeling
日程: 2025年3月18日から3月21日
- 講演(18pL3-2) "ツリーテンソルネットワークを用いた生成モデルにおけるテンソル・ネットワーク最適化"(共同研究者:大久保毅、川島直輝)
- 発表日
- 2025年1月16日
- Seminar
- 第14回学習物理領域セミナー+第66回DLAP
- タイトル
- テンソル木を用いた生成モデルがデータ内の隠れた関係性を抽出する
- 概要
-
ボルンマシンと呼ばれる量子状態の観測結果を基にした生成モデルが提案され、量子コンピュータの応用例として注目されています。一方、ボルンマシンの量子状態をテンソルネットワーク(テンソル分解)で表現することにより、古典計算機でも効率よく実行できる手法も興味深いものです。実際、テンソルネットワークを用いることで、量子回路によるボルンマシンのパラメータ最適化問題を解決できることが示されています。しかし、このアプローチには、テンソルネットワークの構造に性能が大きく依存するという課題があります。我々の最近の研究では、データ内の相互情報量に着目し、ツリーテンソルネットワーク(テンソル木)を用いたボルンマシンにおいて、ネットワーク構造を動的に改善する手法を提案しました。その結果、ボルンマシンの性能向上に加えて、ネットワーク構造からデータ内の関係性を抽出できることが明らかになりました。講演では、ランダムパターン、手書き文字画像、ベイジアンネットワーク、株価の揺らぎなどのデータに適用した実例を紹介します。近年、パラメータ圧縮の観点から機械学習においてテンソル分解が多く用いられており、ネットワーク最適化はその性能向上に貢献する有望なアプローチとして期待できます。
- 文献
- arXiv:2408.10669
- 参照コード
- Adaptive Tensor Tree Generative Modeling
- Date
- December 13th, 2024
- Seminar
- Theory seminar at ISSP, Univ. of Tokyo
- Title
- Tensor tree learns hidden relational structure in data to construct generative models
- Abstract
-
Generative modeling is a significant machine learning technique that constructs the probability distribution of a dataset, owing to its wide range of applications across various problems. Recently, there has been extensive research into generative modeling on quantum computers, referred to as Born machines. This approach utilizes the output of projective measurement of quantum states for stochastic samplings.
We propose a general method for constructing a generative model based on the tree tensor network within the Born machine framework. The core idea is to optimize the tree structure dynamically to minimize the bond mutual information. We demonstrate potential applications with four examples: (1) Random bit sequences with long-range correlation, (2) Images of handwritten digits from the QMNIST dataset, (3) Bayesian networks, (4) Stock price fluctuations in the S&P 500.
Our method significantly enhances performance and reveals hidden relational structures in the target data, paving the way for future improvements and advancements.
- Reference
- arXiv:2408.10669
- Code
- Adaptive Tensor Tree Generative Modeling
- 日程
- 2024年11月15日から11月17日
- 研究会
- TENSOR NETWORK 2024(金沢)
- 講演タイトル
- Tensor tree learns hidden relational structure in data to construct generative models
- 概要
- テンソルネットワークを用いた生成モデルの研究についてレビューを行い、テンソル木を用いたボルン生成マシンを構築する新しい手法を紹介する。キーとなるアイデアは、ボンド相互情報を最小化する木構造を動的に最適化することで、性能を向上させるだけでなく、対象データ中の隠れた関係構造を発見できることである。いくつかの実験例を用いて、その特徴を紹介する。
日程: 2024年9月16日から9月19日
- 講演(17pS101-4) "量子多体系のグラウバーダイナミクスと関連する話題"(シンポジウム「量子多体系におけるダイナミクス研究の進展: 極限宇宙の物理法則を探る」)
- 講演(17pPSB-1) "テンソルネットワークによるモンテカルロ法の高速化"(発表者:馬場謙太郎)
- 講演(17pPSB-54) "テンソルネットワークを用いた強化学習におけるパラメーターの一斉更新へのアプローチ"(発表者:河本佳大)
- 講演(18aE314-8) "ネットワーク構造最適化を含んだツリーテンソルネットワーク法の性能評価"(発表者:引原俊哉、共同研究者:上田宏, 奥西巧一, 西野友年)
- Title
- Tensor tree learns hidden relational structures in data to construct generative models
- Author
- Kenji Harada, Tsuyoshi Okubo, Naoki Kawashima
- Abstract
- Based on the tensor tree network with the Born machine framework, we propose a general method for constructing a generative model by expressing the target distribution function as the quantum wave function amplitude represented by a tensor tree. The key idea is dynamically optimizing the tree structure that minimizes the bond mutual information. The proposed method offers enhanced performance and uncovers hidden relational structures in the target data. We illustrate potential practical applications with four examples: (i) random patterns, (ii) QMNIST hand-written digits, (iii) Bayesian networks, and (iv) the stock price fluctuation pattern in S&P500. In (i) and (ii), strongly correlated variables were concentrated near the center of the network; in (iii), the causality pattern was identified; and, in (iv), a structure corresponding to the eleven sectors emerged.
- Comments
- 9 pages, 3 figures
- Preprint
- arXiv:2408.10669
- Code
- Adaptive Tensor Tree Generative Modeling
- Dates
- March 25-29, 2024
- Conference
- SQAI-NCTS Workshop on Tensor Network and Quantum Embedding (Hongo Campus, The University of Tokyo)
- Title
- Optimizing the structure of tree tensor network for quantum generative modeling using mutual information-based approach
- Abstract
- Generative modeling is a crucial task in the field of machine learning. Recently, there have been several proposals for generative models on quantum devices. We can efficiently optimize generative models defined by tensor network states, but their performance largely depends on the geometrical structure of the tensor network. To tackle this issue, we have proposed an optimization method for the network structure in the tree tensor network class, based on the least mutual information principle. Generative modeling with an optimized network structure has better performance than a fixed network structure. Moreover, by embedding data dependencies into the tree structure based on the least mutual information principle, we can geometrically represent the correlations in the data.
TOPICS
ACTIVITY
学術変革領域(A)極限宇宙の物理法則を創る-量子情報で拓く時空と物質の新しいパラダイム
ABOUT
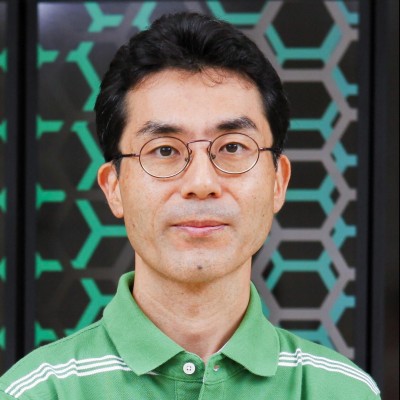
原田健自
(
Kenji Harada
)
京都大学大学院情報学研究科
助教
harada.kenji.8e@kyoto-u.ac.jp
京都市左京区吉田本町 京都大学吉田キャンパス 総合研究8号館203号室
Map
(No.59)
統計物理学と情報論的視点を融合した最先端の計算手法とスーパーコンピュータのパワーを組み合わせ、相互作用する多体系と情報科学における未解決問題に先端的に取り組んでいます。