More is different.
God is in the details.
NEWS
- Title
- Tensor tree learns hidden relational structures in data to construct generative models
- Author
- Kenji Harada, Tsuyoshi Okubo, Naoki Kawashima
- Abstract
- Based on the tensor tree network with the Born machine framework, we propose a general method for constructing a generative model by expressing the target distribution function as the amplitude of the quantum wave function represented by a tensor tree. The key idea is dynamically optimizing the tree structure that minimizes the bond mutual information. The proposed method offers enhanced performance and uncovers hidden relational structures in the target data. We illustrate potential practical applications with four examples: (i) random patterns, (ii) QMNIST handwritten digits, (iii) Bayesian networks, and (iv) the pattern of stock price fluctuation pattern in S\&P500. In (i) and (ii), the strongly correlated variables were concentrated near the center of the network; in (iii), the causality pattern was identified; and in (iv), a structure corresponding to the eleven sectors emerged.
- Comments
- 10 pages, 3 figures
- Citation
- Kenji Harada, Tsuyoshi Okubo, and Naoki Kawashima, Machine Learning: Science and Technology 6 025002(2025)
- DOI
- 10.1088/2632-2153/adc2c7
- Code
- Adaptive Tensor Tree Generative Modeling
- Date
- December 13th, 2024
- Seminar
- Theory seminar at ISSP, Univ. of Tokyo
- Title
- Tensor tree learns hidden relational structure in data to construct generative models
- Abstract
-
Generative modeling is a significant machine learning technique that constructs the probability distribution of a dataset, owing to its wide range of applications across various problems. Recently, there has been extensive research into generative modeling on quantum computers, referred to as Born machines. This approach utilizes the output of projective measurement of quantum states for stochastic samplings.
We propose a general method for constructing a generative model based on the tree tensor network within the Born machine framework. The core idea is to optimize the tree structure dynamically to minimize the bond mutual information. We demonstrate potential applications with four examples: (1) Random bit sequences with long-range correlation, (2) Images of handwritten digits from the QMNIST dataset, (3) Bayesian networks, (4) Stock price fluctuations in the S&P 500.
Our method significantly enhances performance and reveals hidden relational structures in the target data, paving the way for future improvements and advancements.
- Reference
- arXiv:2408.10669
- Code
- Adaptive Tensor Tree Generative Modeling
- Title
- Tensor tree learns hidden relational structures in data to construct generative models
- Author
- Kenji Harada, Tsuyoshi Okubo, Naoki Kawashima
- Abstract
- Based on the tensor tree network with the Born machine framework, we propose a general method for constructing a generative model by expressing the target distribution function as the quantum wave function amplitude represented by a tensor tree. The key idea is dynamically optimizing the tree structure that minimizes the bond mutual information. The proposed method offers enhanced performance and uncovers hidden relational structures in the target data. We illustrate potential practical applications with four examples: (i) random patterns, (ii) QMNIST hand-written digits, (iii) Bayesian networks, and (iv) the stock price fluctuation pattern in S&P500. In (i) and (ii), strongly correlated variables were concentrated near the center of the network; in (iii), the causality pattern was identified; and, in (iv), a structure corresponding to the eleven sectors emerged.
- Comments
- 9 pages, 3 figures
- Preprint
- arXiv:2408.10669
- Code
- Adaptive Tensor Tree Generative Modeling
- Dates
- March 25-29, 2024
- Conference
- SQAI-NCTS Workshop on Tensor Network and Quantum Embedding (Hongo Campus, The University of Tokyo)
- Title
- Optimizing the structure of tree tensor network for quantum generative modeling using mutual information-based approach
- Abstract
- Generative modeling is a crucial task in the field of machine learning. Recently, there have been several proposals for generative models on quantum devices. We can efficiently optimize generative models defined by tensor network states, but their performance largely depends on the geometrical structure of the tensor network. To tackle this issue, we have proposed an optimization method for the network structure in the tree tensor network class, based on the least mutual information principle. Generative modeling with an optimized network structure has better performance than a fixed network structure. Moreover, by embedding data dependencies into the tree structure based on the least mutual information principle, we can geometrically represent the correlations in the data.
- Book title
- Advanced Mathematical Science for Mobility Society
- Editors
- Kazushi Ikeda, Yoshiumi Kawamura, Kazuhisa Makino, Satoshi Tsujimoto, Nobuo Yamashita, Shintaro Yoshizawa, Hanna Sumita
- Publisher
- Springer Singapore
- Reference
- ISBN 978-981-99-9771-8 ISBN 978-981-99-9772-5 (eBook)
- Title
- Chapter 5 "Application of Tensor Network Formalism for Processing Tensor Data"
- Authors
- Kenji Harada, Hiroaki Matsueda, and Tsuyoshi Okubo
- Web page
- Open access
- Date
- Jan 26, 2024
- Conference
- 2024 Annual Meeting of the Physical Society of Taiwan, Topical Symposia:Many-body systems and advanced numerical methods
- Title
- Optimizing tensor network structure
TOPICS
Toolkit of Bayesian Scaling Analysis
Reference application software of a new scaling analysis method of critical phenomena based on Bayesian inference.
To demo To detailsToolkit of Adaptive Tensor Tree Generative Modeling
Reference application software of adaptive tensor tree generative modeling.
To GitHubMonte Carlo simulations
This demonstration shows a Monte Carlo simulation of the two-dimensional Ising model by three algorithms: Metropolis, Swendsen-Wang, and Wolff algorithms.
To demoABOUT
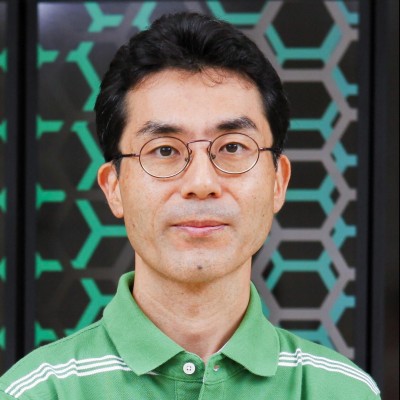
Kenji Harada
(
原田健自
)
Assistant Professor,
Graduate School of Informatics, Kyoto University, Japan.
harada.kenji.8e@kyoto-u.ac.jp
Room 203, Research Bldg. No.8, Yoshida Campus, Kyoto Univ., Kyoto, 606-8501, Japan.
Map (No.59)